3D Geological Image Synthesis from 2D Examples using Generative Adversarial Networks
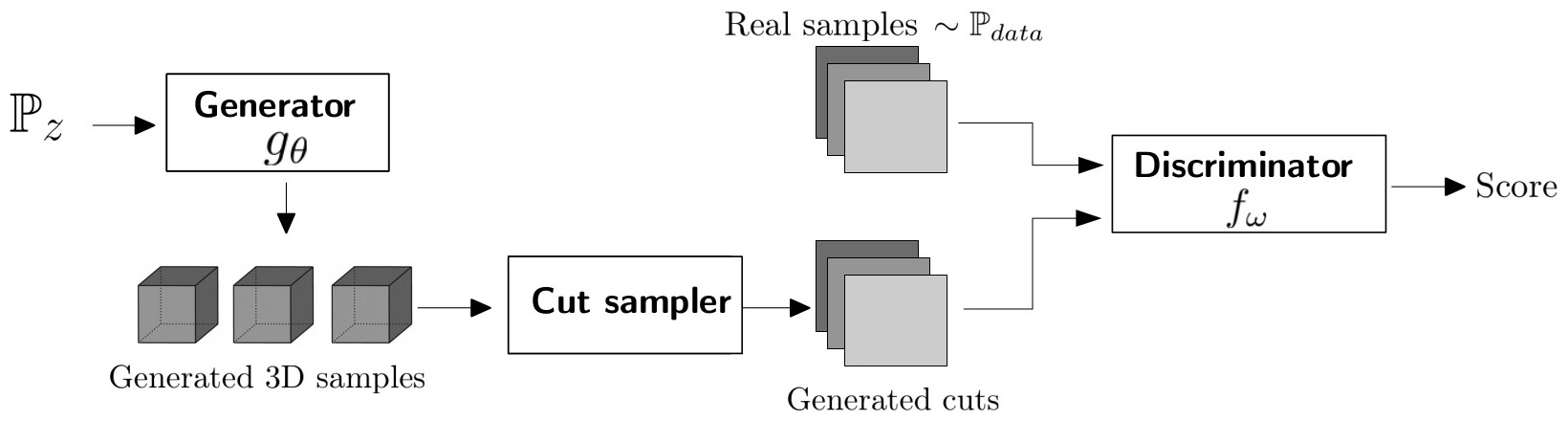
Generative Adversarial Networks (GAN) are becoming an alternative to Multiple-point Statistics (MPS) techniques to generate stochastic fields from training images. But a difficulty for all the training image based techniques (including GAN and MPS) is to generate 3D fields when only 2D training data sets are available. In this paper, we introduce a novel approach called Dimension Augmenter GAN (DiAGAN) enabling GANs to generate 3D fields from 2D examples. The method is simple to implement and is based on the introduction of a random cut sampling step between the generator and the discriminator of a standard GAN. Numerical experiments show that the proposed approach provides an efficient solution to this long lasting problem.
A preliminary version of this work was presented at the Petroleum Geostatistics Conference in 2019.